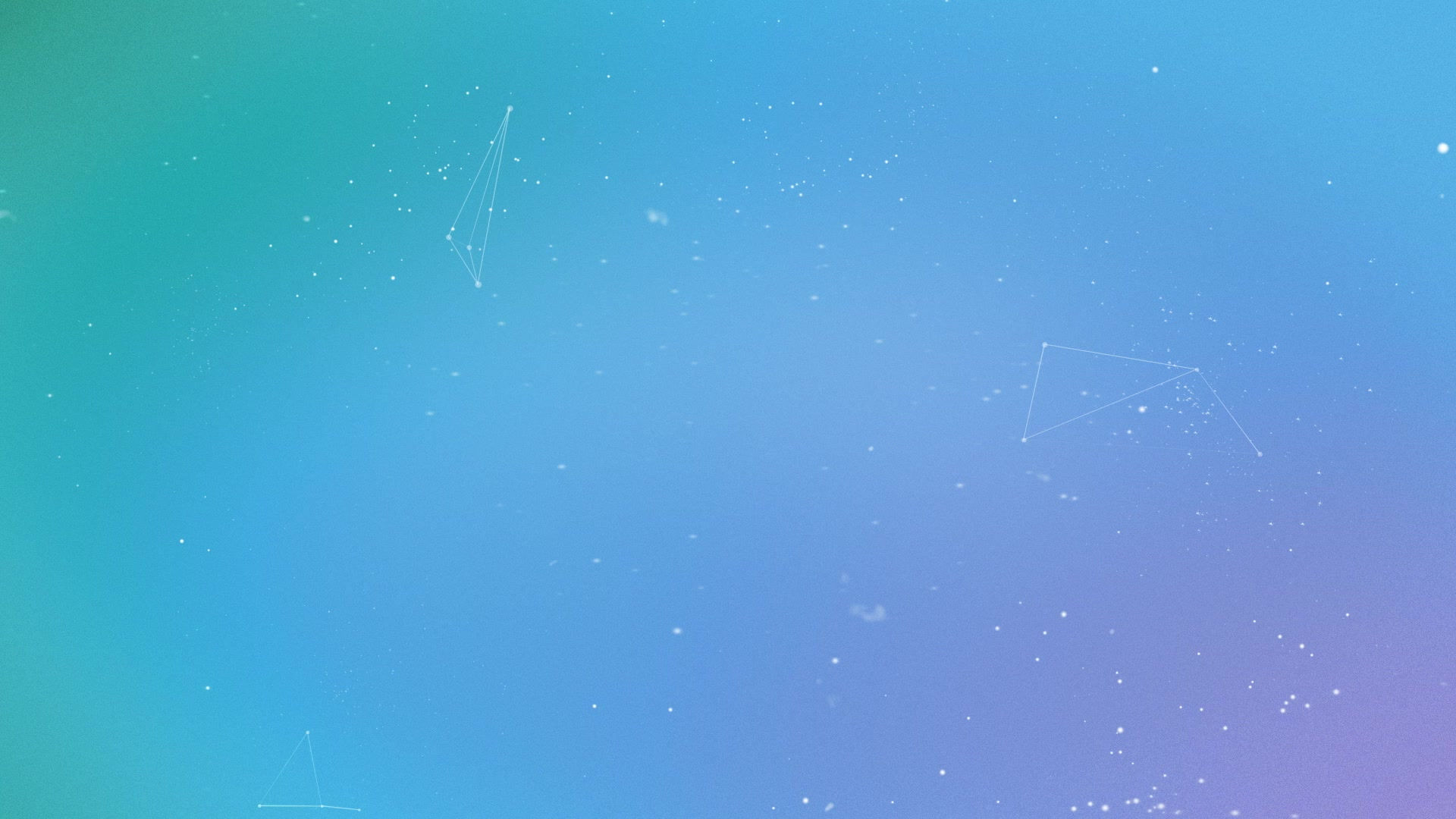
Multi-omics
Epigenomics
(1) Tracking stem cell differentiation and predicting of cell pluripotency
To understand the molecular mechanisms associated with cellular differentiation, we focus on research on dynamic DNA methylation during cell differentiation and self-renewal. In this research, we perform tracing dynamic changes of DNA methylation at single cell resolution and constructing computational models for predicting cell pluripotency by methylome sequencing data including other omics-data. Here, we approach the computational modeling method for multivariate and large scale numerical matrix analysis such as regression analysis. Finally, we aim to identify molecular biomarkers of cell differentiation for understanding dynamic cell heterogeneity during epigenetic reprograming which can be candidates of regenerative medicines.
(2) Computational modeling for biological age prediction and identifying epigenetic bio-marker aging control
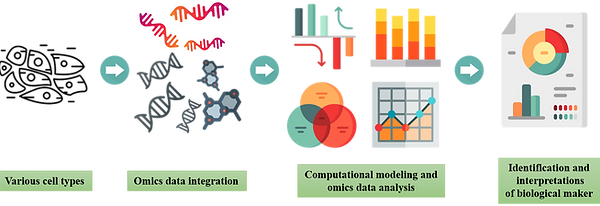
Aging involves various types of biological pathways and finding aging-related biomarkers, such as telomere length, gene expression provide insight into age-associated diseases. In this research, we would like to construct age prediction models using DNA methylation data and to find aging-related epigenetic markers in multiple tissues. For model construction, we tries to overcome high dimensional problems in biological data by applying regularization technique and various machine learning algorithms. Furthermore, we try to make comparative analysis using various omics data including m6A (N6-Methyladenosine) RNA immunoprecipitation approach followed by high-throughput sequencing (MeRIP-seq) between old and young mice. So, our aims of this research are finding aging-related epigenetic markers and understanding the mechanisms of human aging

Cancer
(1) Cancer Prognosis Prediction
Cancer prognosis is important to decide which treatment should be applied to the patient. If we are able to predict the accurate prognosis of cancer patients, we can recommend and offer appropriate therapies or drugs to patients. Recently, many researchers are trying to construct a deep learning based prognosis prediction model and their model shows better performance than conventional machine learning methods. However, they still could not overcome main challenges in this field which is high dimension, small sample size dataset. To overcome these challenges, we are focusing on constructing accurate and robust prediction model with reducing redundant model parameters and adding interpretable structure in the model. We anticipate more robust and powerful features are selected for prognosis prediction.

(2) Cancer Metabolic Biomarker Identification
Cancer cells have unique characteristics for survival such as abnormal proliferation, metastasis, angiogenesis and apoptosis. It evokes alteration at multiple levels; genome, transcriptome, epigenome, proteome and metabolome. Confirming the mutation of upper level biomolecules is important and can be the basis of treatment, but there are some cost limitations. To diagnose cancer easier, we focus on metabolomics which is research about the profiling of metabolites. The researchers in our lab not only use the traditional statistical analysis but also apply the machine learning methods for improving the accuracy of cancer diagnosis. We expect those differentiated metabolites to give the insight of cancer biology more than just a cancer diagnostic biomarker.
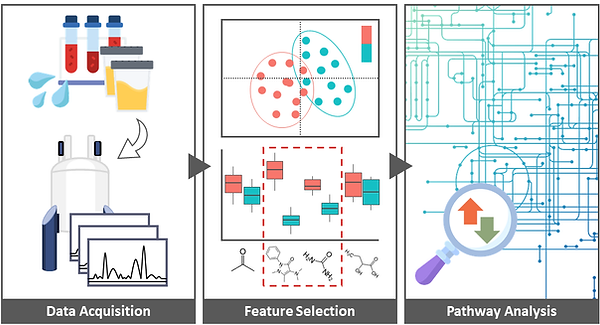
(3) Cancer-Drug Response Prediction
Cancer patients show different responses to a drug; someone needs only a small dose of drug while it doesn’t work for other one. So many experimental trials were performed to predict the response of a drug on a cell line by using in vivo and in vitro methods. But those methods, like a xenograft model, are time-consuming jobs with high costs. Computational prediction methods with deep learning can be a good solution for this problem. In fact, many deep learning-based cancer-drug response prediction methods have been published. But those models showed relatively low performance, and also were not interpretable. The researchers in our lab are developing the model for cancer-drug response prediction which have high prediction performance and interpretability. By using this model, a new compound can be identified as the anti-cancer drug candidate, and the MoA of cancer-drug response also can be identified.
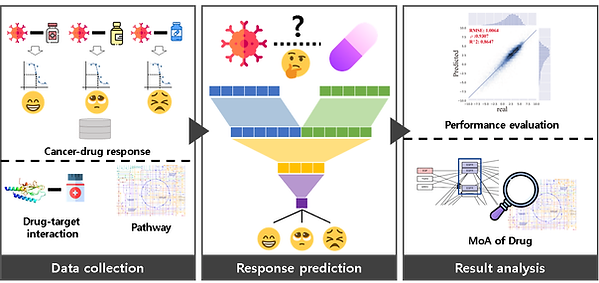